<目次>
(1) Azure Machine LearningでDataStoreを作成してAzure Storage File Shares(ファイル共有)のデータを操作する方法
(0-1) やりたいこと
(1-0) STEP0:前提条件(例)
(1-1) STEP1:ワークスペースへの接続
(1-2) STEP2:DataStoreの生成
(1-3) STEP3:DataStoreを経由したデータアクセス処理の記述
(1-4) STEP4:実行と結果確認
(1) Azure Machine LearningでDataStoreを作成してAzure Storage File Shares(ファイル共有)のデータを操作する方法
(0-1) やりたいこと
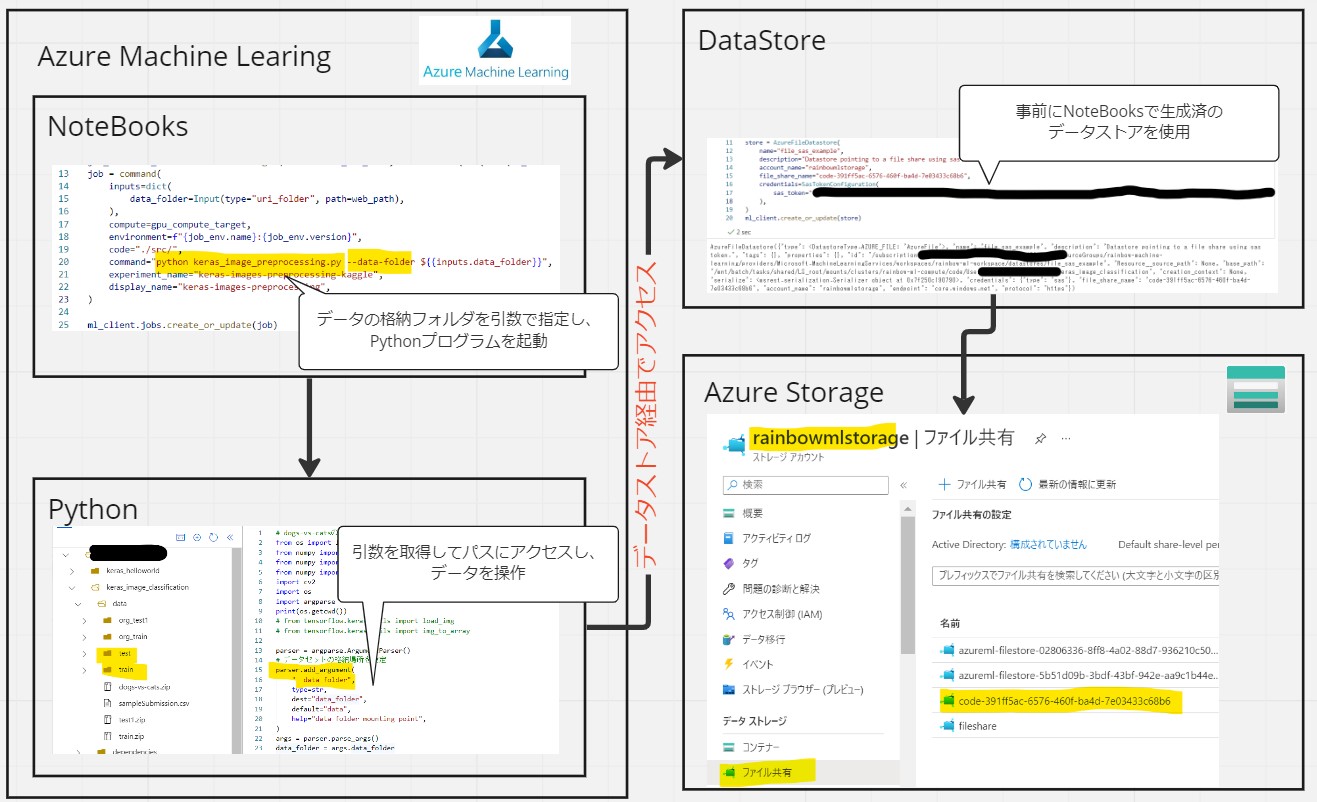
・MS公式サイトによると、DataStoreは既存のStorage AccountをAzure Machine Learningで使えるようにするための『紐づけ設定』の様な位置づけです。
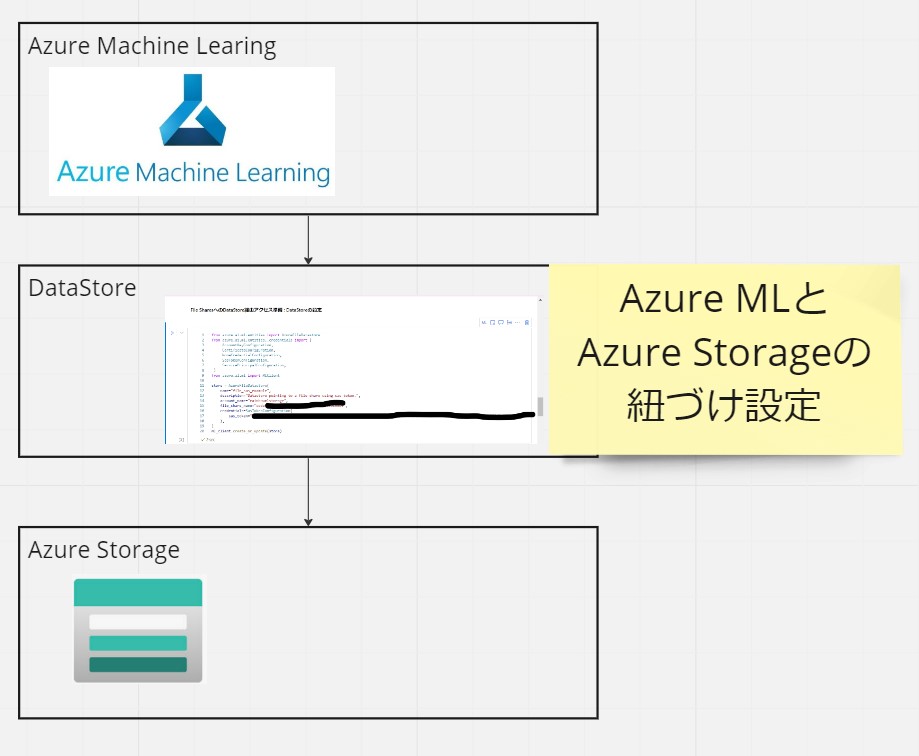
(1-0) STEP0:前提条件(例)
●STEP0-1:Azure Machine Learning
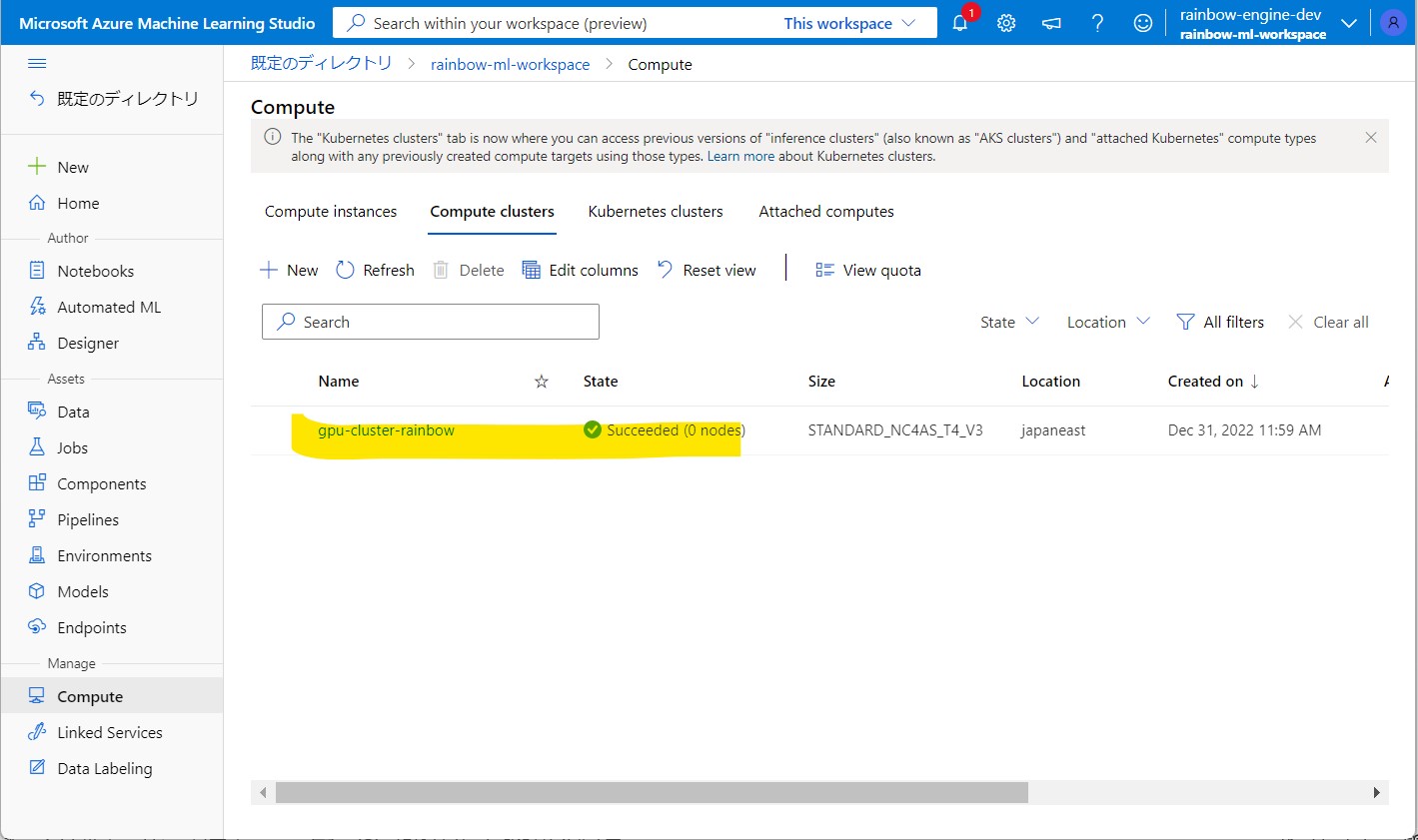
●STEP0-2:Azure Storage
(1-1) STEP1:ワークスペースへの接続
# Handle to the workspace from azure.ai.ml import MLClient # Authentication package from azure.identity import DefaultAzureCredential credential = DefaultAzureCredential()
(セル値1-2)
# Get a handle to the workspace ml_client = MLClient( credential=credential, subscription_id="[サブスクリプションID]", resource_group_name="[Azure Machine Learningのリソースグループ名]", workspace_name="[Azure Machine Learningのワークスペース名]", )
(1-2) STEP2:DataStoreの生成
●STEP2-1:Azure Storage側で、SASトークンを生成
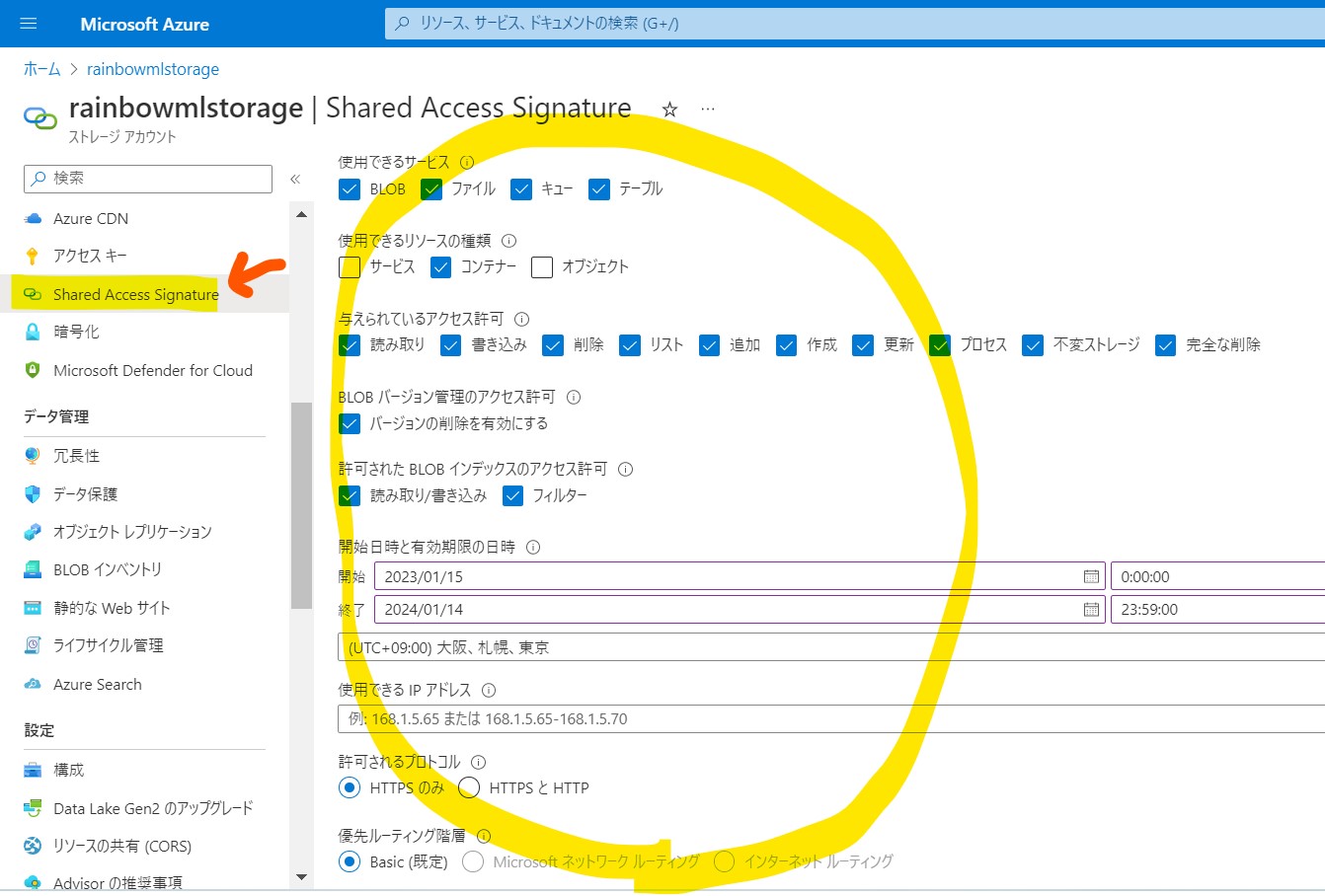
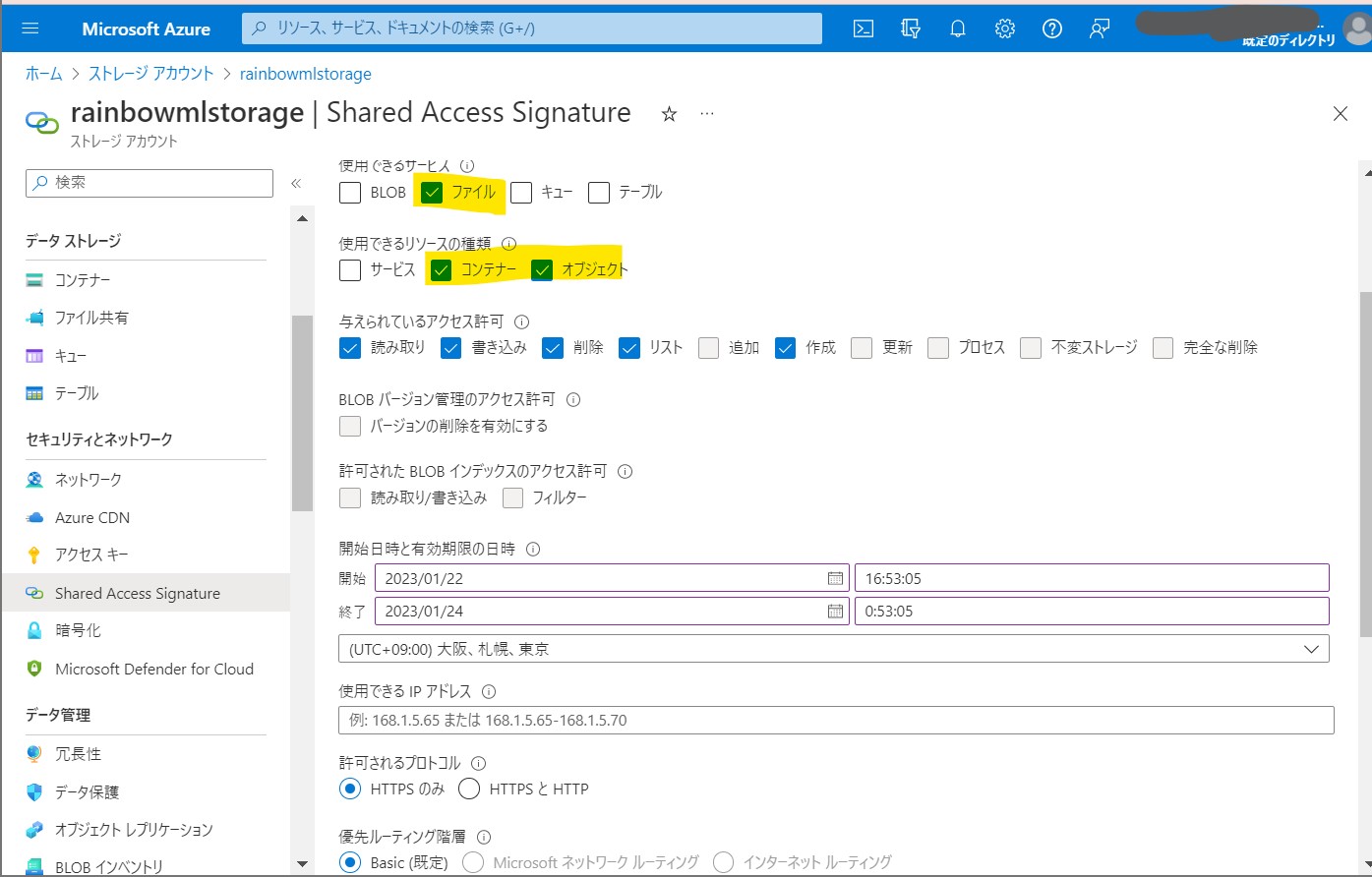
(補足)設定の理由
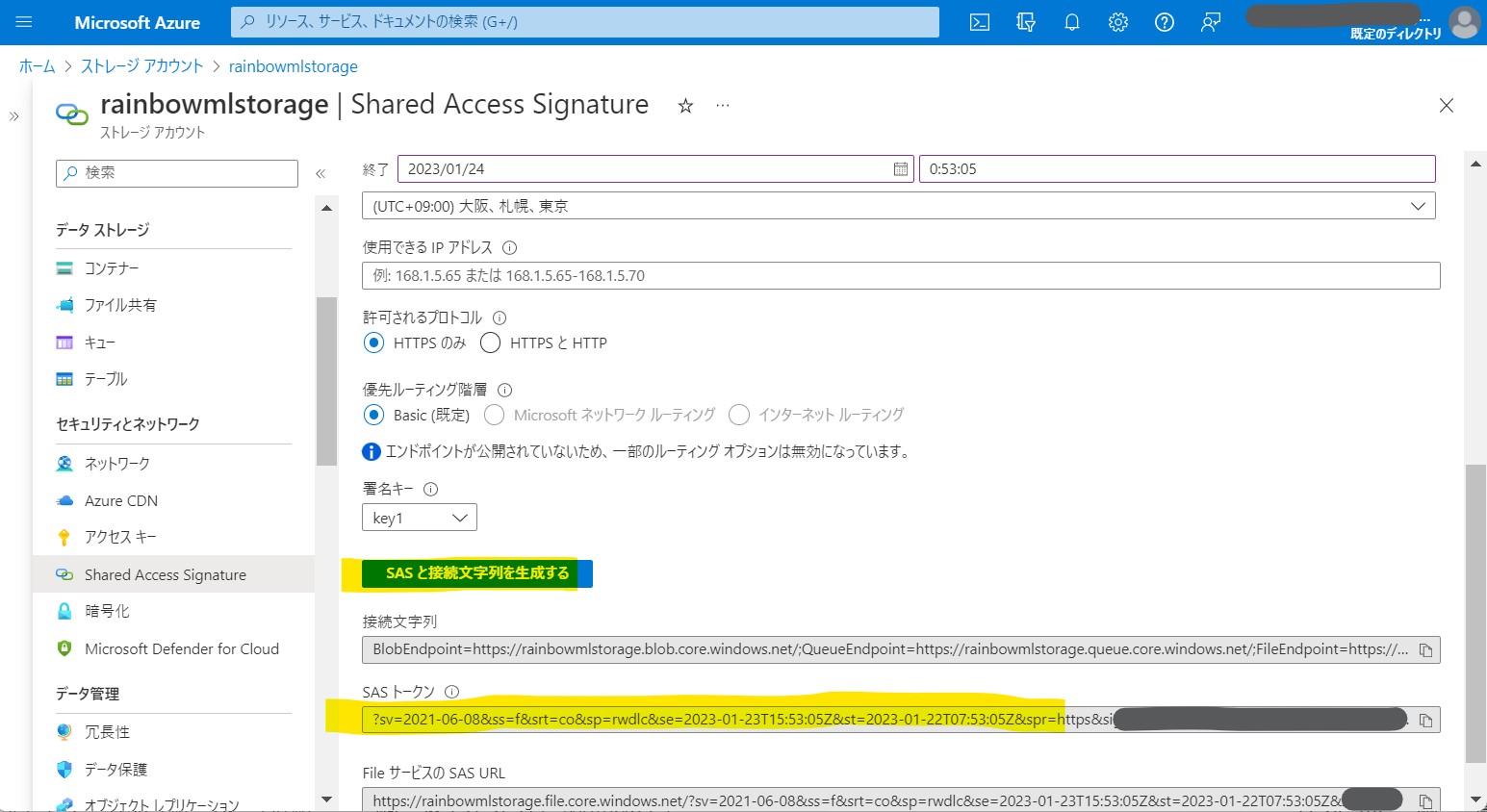
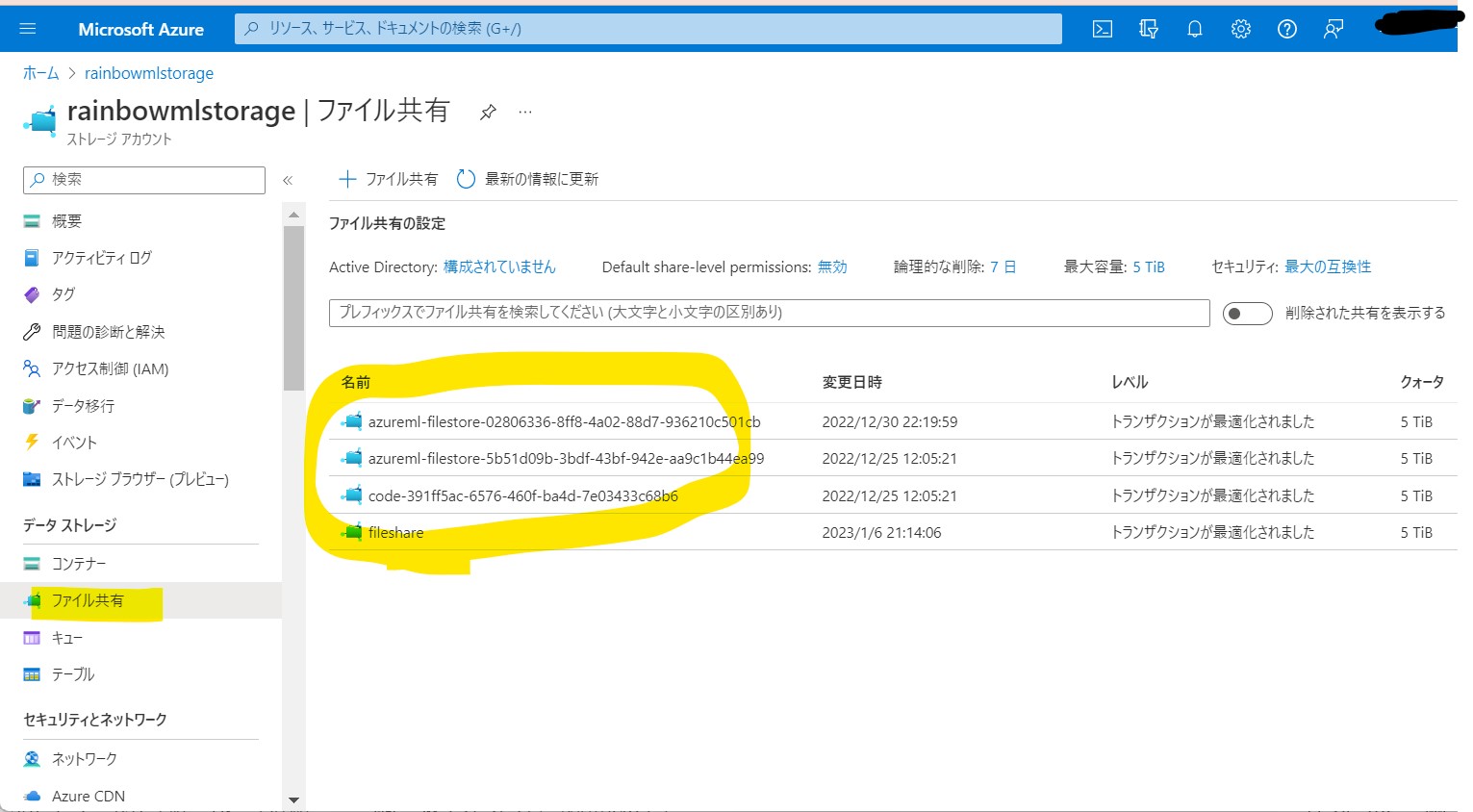
●STEP2-2:Azure Machine LearningのNotebooksの実装
from azure.ai.ml.entities import AzureFileDatastore from azure.ai.ml.entities._credentials import ( AccountKeyConfiguration, CertificateConfiguration, NoneCredentialConfiguration, SasTokenConfiguration, ServicePrincipalConfiguration, ) from azure.ai.ml import MLClient store = AzureFileDatastore( name="file_sas_example", description="Datastore pointing to a file share using sas token.", account_name="[ご自身のストレージアカウント名]", file_share_name="[ご自身のストレージアカウントのファイル共有名]", credentials=SasTokenConfiguration( sas_token="[ご自身のSAS Token]" ), ) ml_client.create_or_update(store)
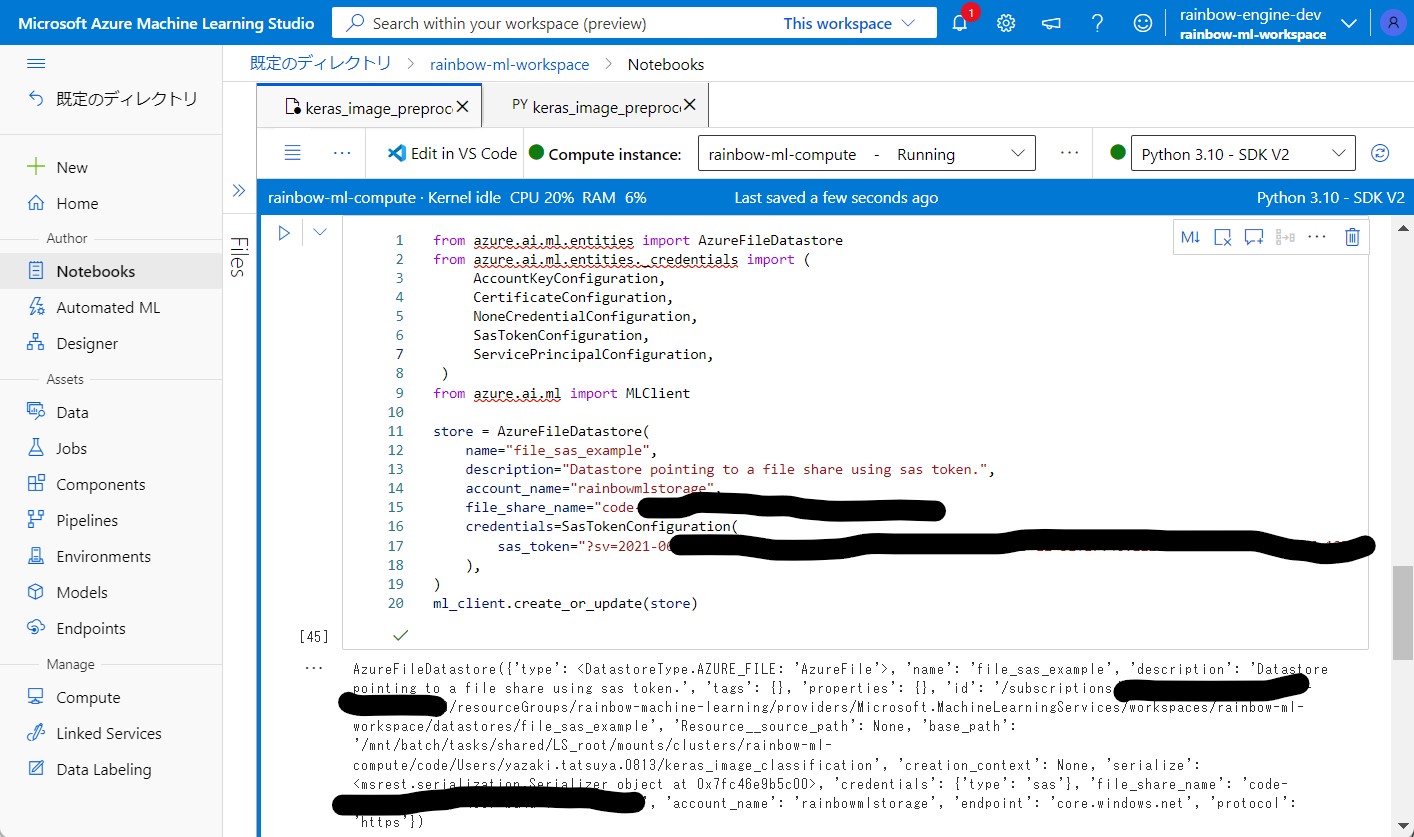
(結果例)
AzureFileDatastore({ 'type': , 'name': 'file_sas_example', 'description': 'Datastore pointing to a file share using sas token.', 'tags': {}, 'properties': {}, 'id': '/subscriptions/xxxxx/resourceGroups/rainbow-machine-learning/providers/Microsoft.MachineLearningServices/workspaces/rainbow-ml-workspace/datastores/file_sas_example', 'Resource__source_path': None, 'base_path': '/mnt/batch/tasks/shared/LS_root/mounts/clusters/rainbow-ml-compute/code/Users/xxxxx/keras_image_classification', 'creation_context': None, 'serialize': , 'credentials': {'type': 'sas'}, 'file_share_name': 'code-391ff5ac-6576-460f-ba4d-7e03433c68b6', 'account_name': 'rainbowmlstorage', 'endpoint': 'core.windows.net', 'protocol': 'https'})
(1-3) STEP3:DataStoreを経由したデータアクセス処理の記述
●STEP3-1:Azure Machine LearningのNotebooksの実装
from azure.ai.ml import command from azure.ai.ml import UserIdentityConfiguration from azure.ai.ml import Input gpu_compute_target = "[ご自身のCompute clusters名]" custom_env_name = "keras-env" web_path = "azureml://datastores/file_sas_example/paths/Users/[ご自身のデータ格納先パス]" # ジョブの最新環境を取得 job_env = ml_client.environments.get(name=custom_env_name, version=str(len(list(ml_client.environments.list(name=custom_env_name))))) # ジョブの定義 job = command( # 入力パラメータの設定 inputs=dict( data_folder=Input(type="uri_folder", path=web_path), ), # CPU/GPUリソース compute=gpu_compute_target, # 実行環境 environment=f"{job_env.name}:{job_env.version}", # 実行対象モジュールの格納先 code="./src/", # 実行対象モジュールと引数 command="python datastore_test_get_image.py --data-folder ${{inputs.data_folder}}", experiment_name="datastore_test_get_image", display_name="datastore_test_get_image", ) # ジョブの実行 ml_client.jobs.create_or_update(job)
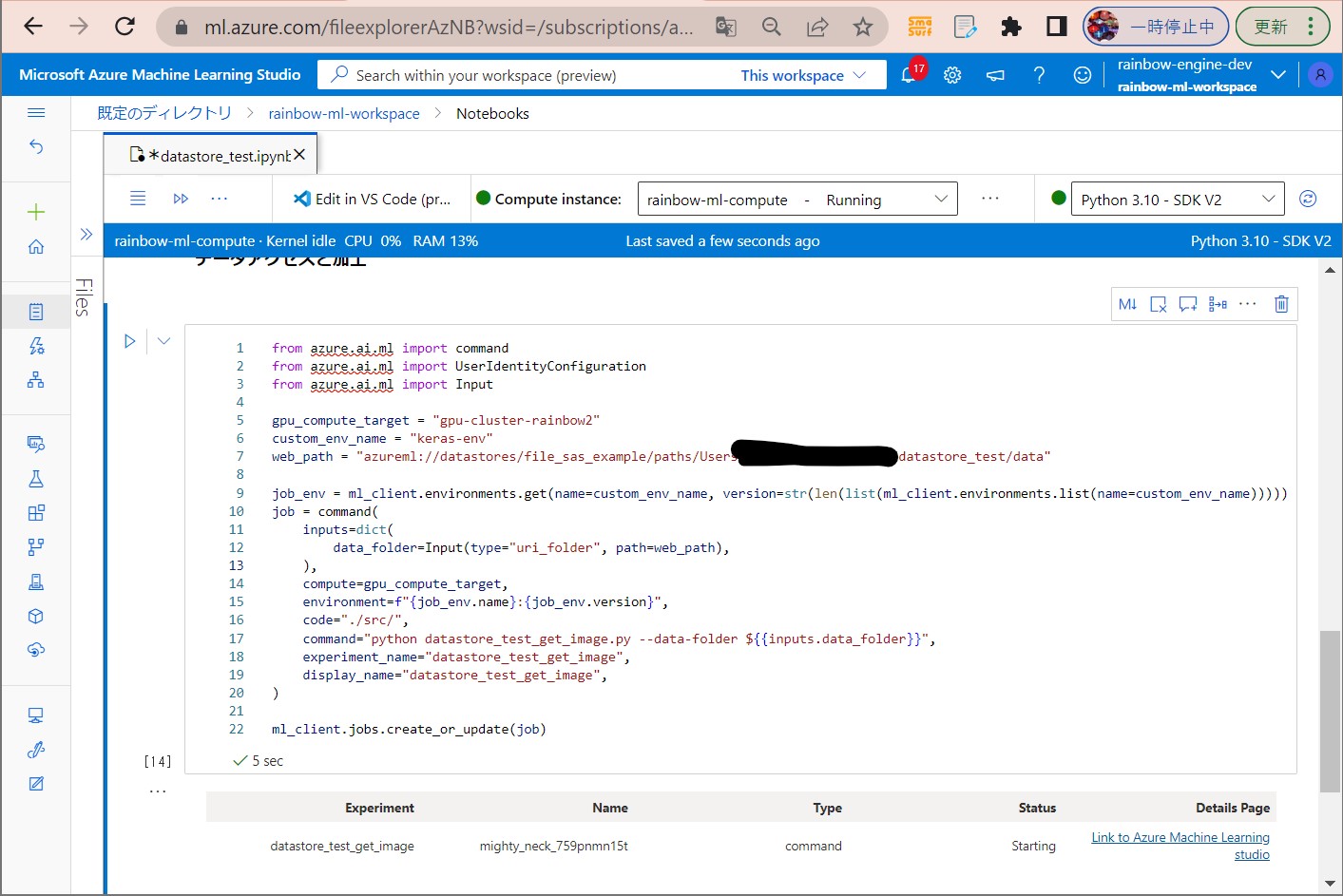
●STEP3-2:NoteBooks経由で呼び出すPythonプログラムの実装
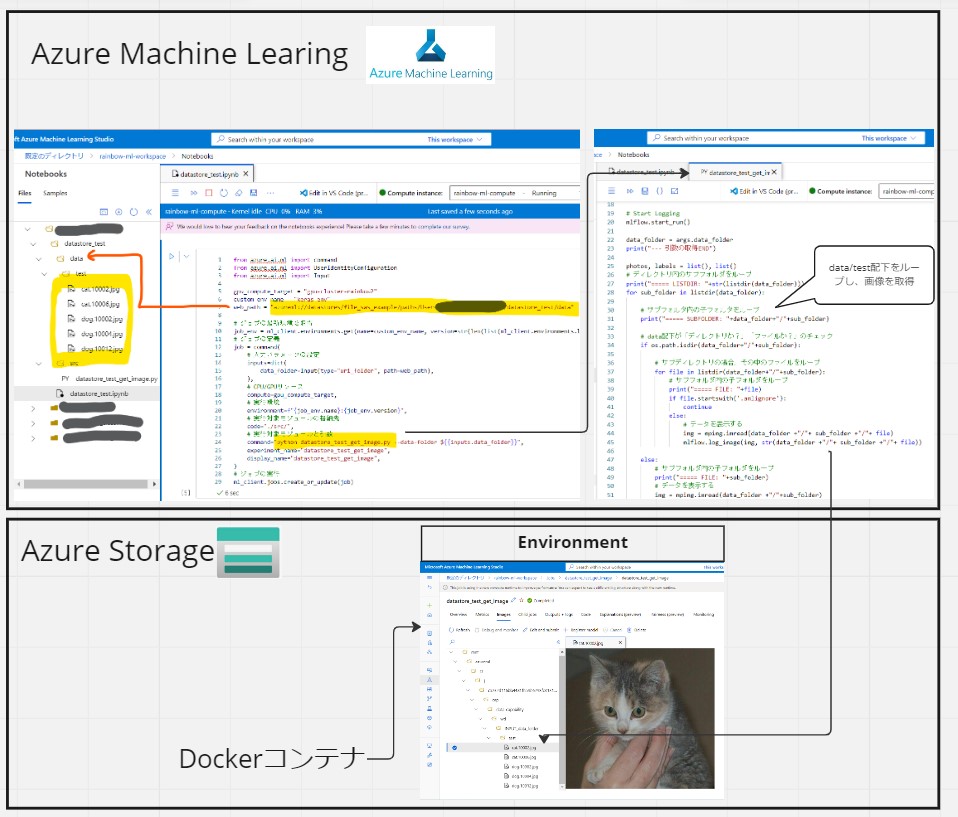
from os import listdir import os import argparse import matplotlib.image as mpimg import mlflow print("--- 引数の取得START") parser = argparse.ArgumentParser() # データセットの格納場所を設定 parser.add_argument( "--data-folder", type=str, dest="data_folder", default="data", help="data folder mounting point", ) args = parser.parse_args() # Start Logging mlflow.start_run() data_folder = args.data_folder print("--- 引数の取得END") photos, labels = list(), list() # ディレクトリ内のサブフォルダをループ print("===== LISTDIR: "+str(listdir(data_folder))) for sub_folder in listdir(data_folder): # サブフォルダ内の子フォルダをループ print("===== SUBFOLDER: "+data_folder+"/"+sub_folder) # data配下が「ディレクトリか?」「ファイルか?」のチェック if os.path.isdir(data_folder+"/"+sub_folder): # サブディレクトリの場合、その中のファイルをループ for file in listdir(data_folder+"/"+sub_folder): # サブフォルダ内の子フォルダをループ print("===== FILE: "+file) if file.startswith('.amlignore'): continue else: # データを保存する img = mpimg.imread(data_folder +"/"+ sub_folder +"/"+ file) mlflow.log_image(img, str(data_folder +"/"+ sub_folder +"/"+ file)) else: # サブフォルダ内の子フォルダをループ print("===== FILE: "+sub_folder) # データを保存する img = mpimg.imread(data_folder +"/"+sub_folder) mlflow.log_image(img, str(data_folder +"/"+sub_folder)) mlflow.end_run()
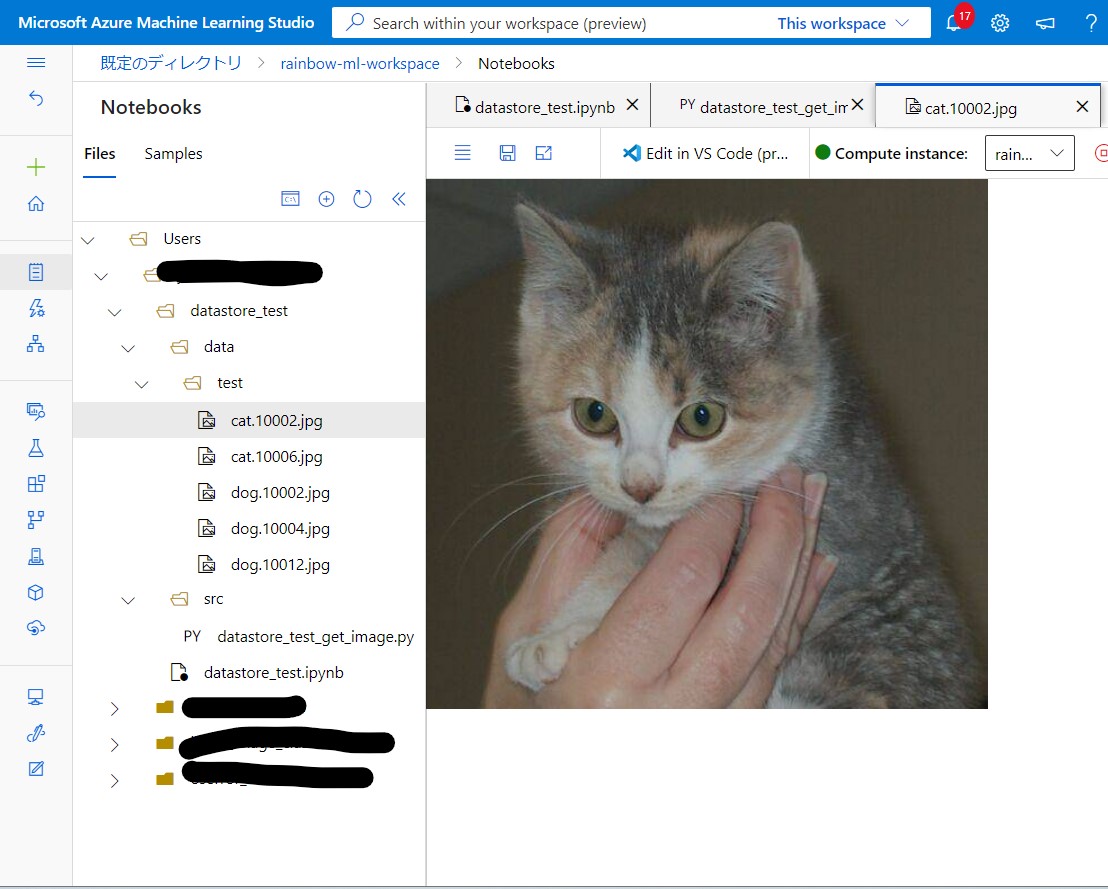
(1-4) STEP4:実行と結果確認
●STEP4-1:セルを順番に実行
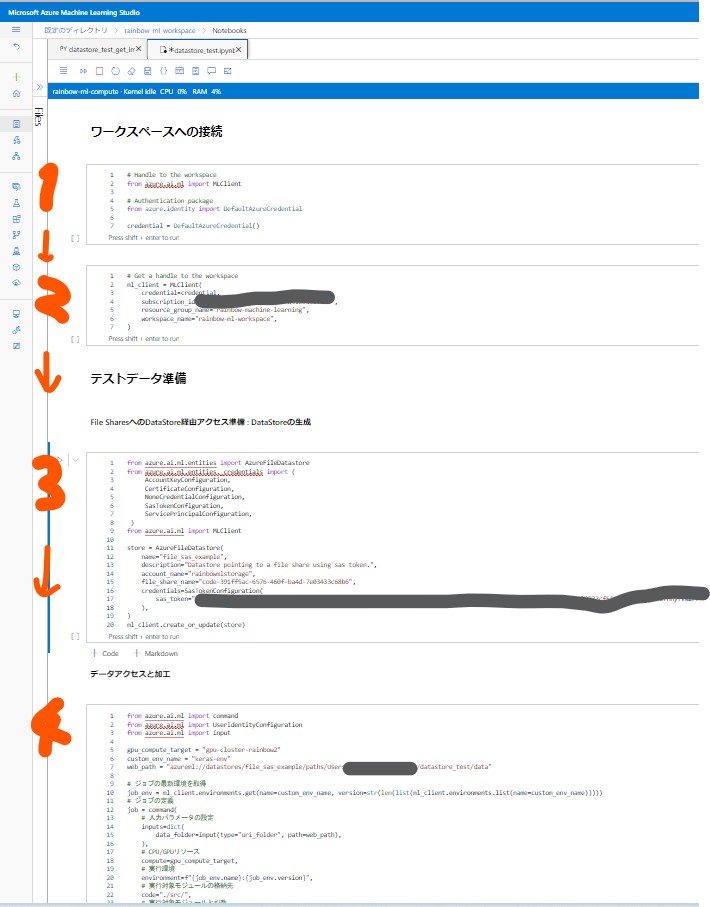
●STEP4-2:結果確認
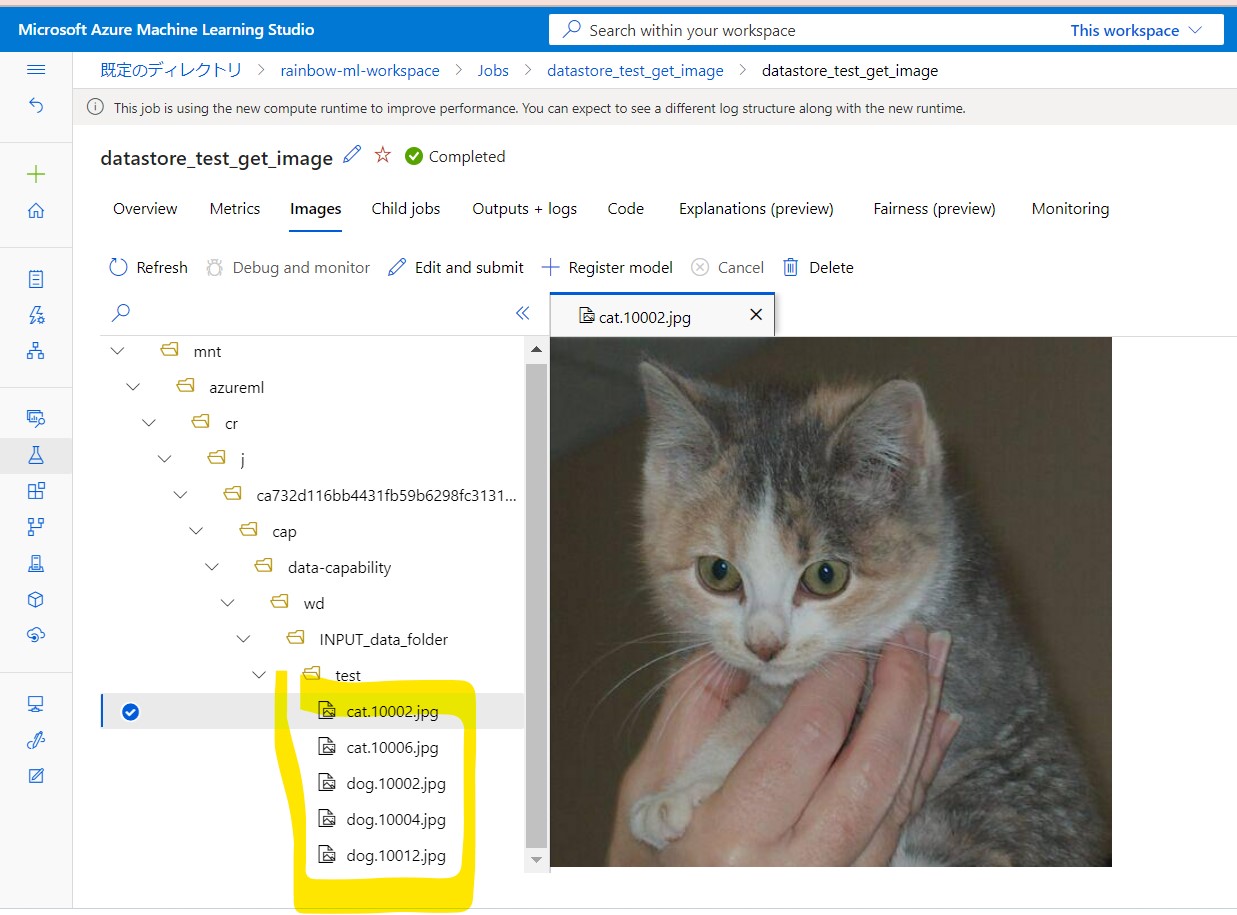