<目次>
(1) Azure Machine LearningからAzure StorageのFile Shares(ファイル共有)のデータにアクセスする方法
やりたいこと
(1-1) STEP1:前提条件(例)
(1-2) STEP2:「Manage Indentity」を持つCompute Clusterの設定
(1-3) STEP3:File Sharesへのデータアクセス処理の記述&実行
(1-4) STEP4:実行と結果確認
(1) Azure Machine LearningからAzure StorageのFile Shares(ファイル共有)のデータにアクセスする方法
やりたいこと
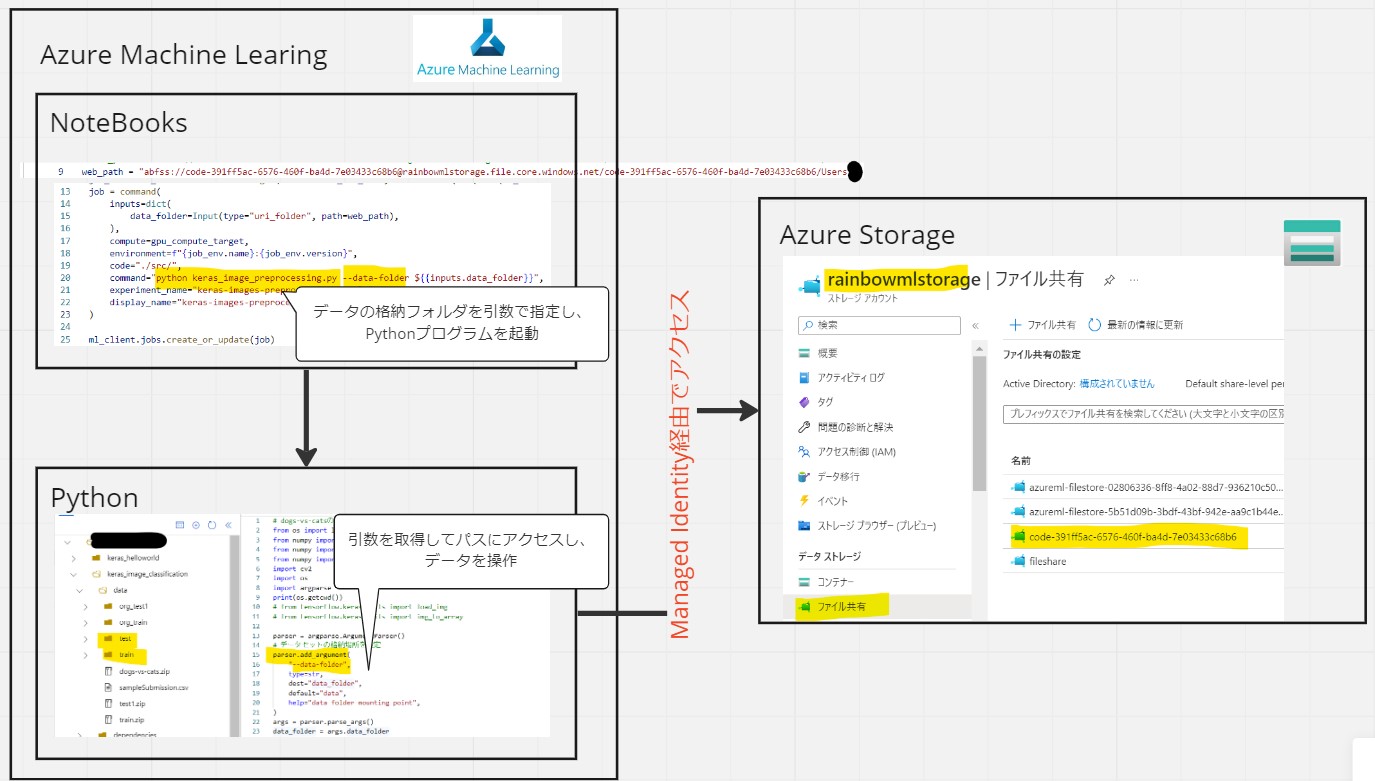
(1-1) STEP1:前提条件(例)
●STEP1-1:Azure Machine Learning
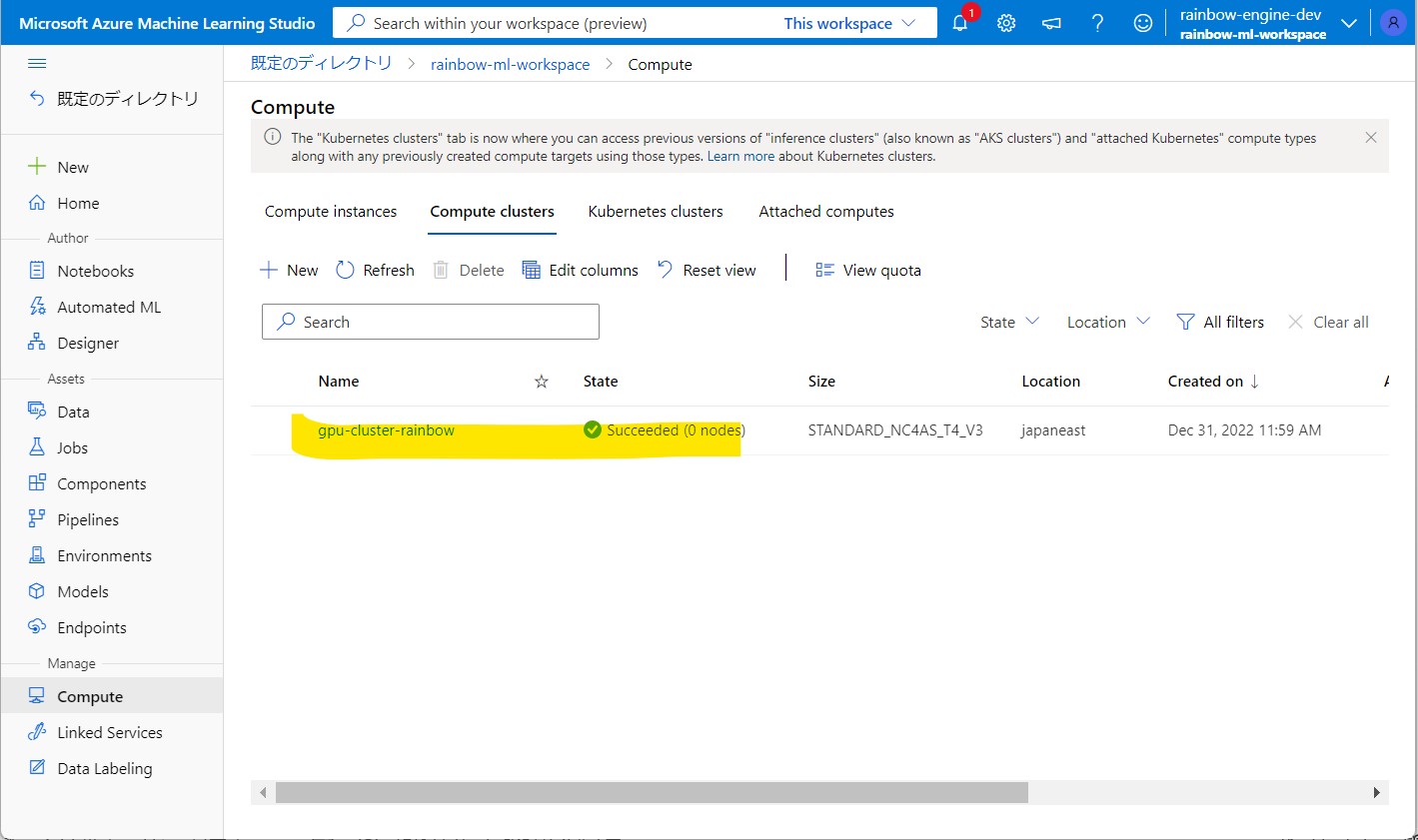
●STEP1-2:Azure Storage
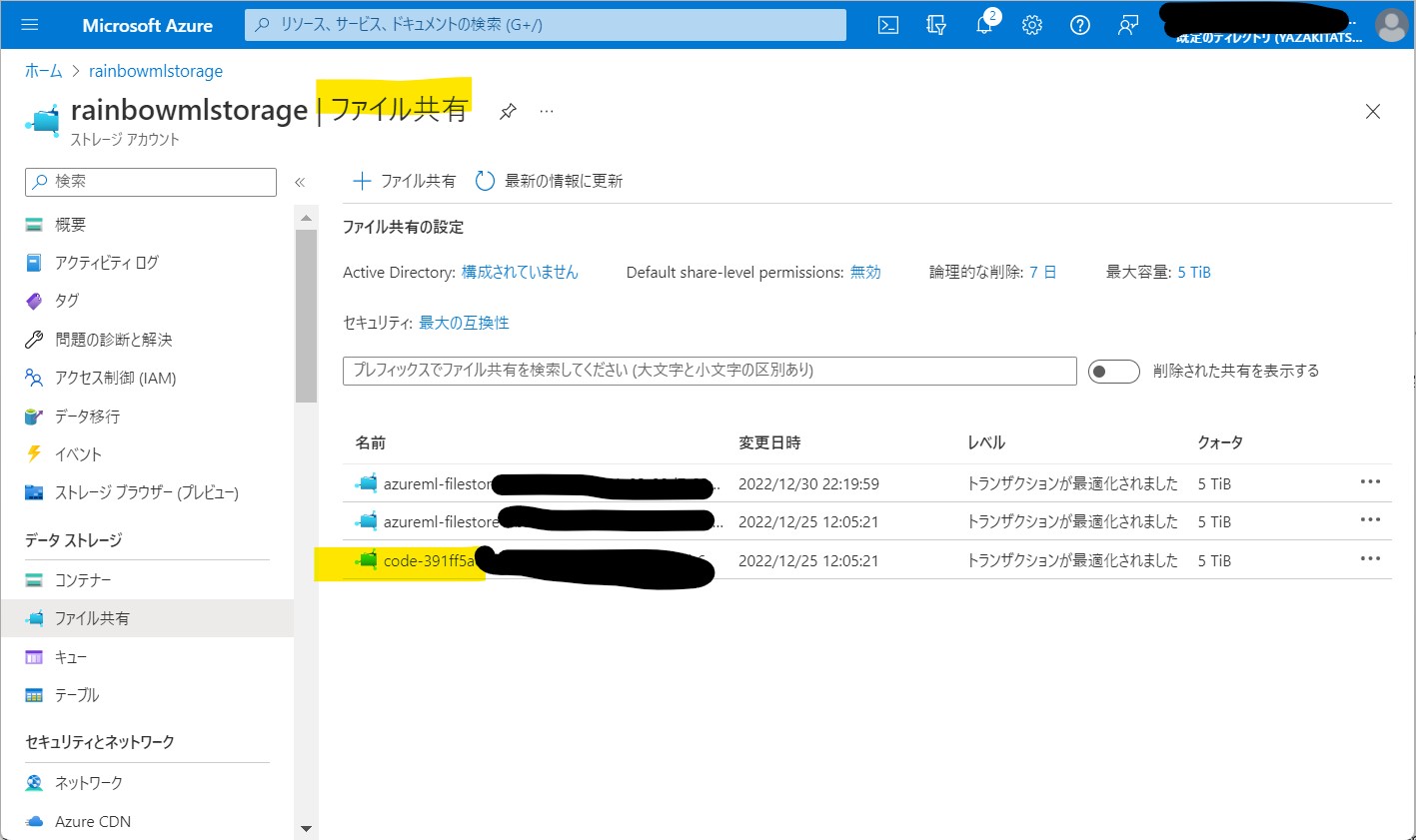
(1-2) STEP2:「Manage Indentity」を持つCompute Clusterの設定
ラベル | 説明 |
設定操作 | Azure Machine LearningのCompute clusterに対して「Manage Identity」の設定を行います。 |
サポートするアイデンティティの種類 | 「one system-assigned identity」または「multiple user-assigned identities」をサポートします。 |
デフォルト設定 | デフォルトは「one system-assigned identity」を使用します。 |
用途説明 | Azure MLにおけるストレージのマウント、データストア、コンテナレジストリ等で使用されます。 |
●STEP2-1:Managed Identityの作成
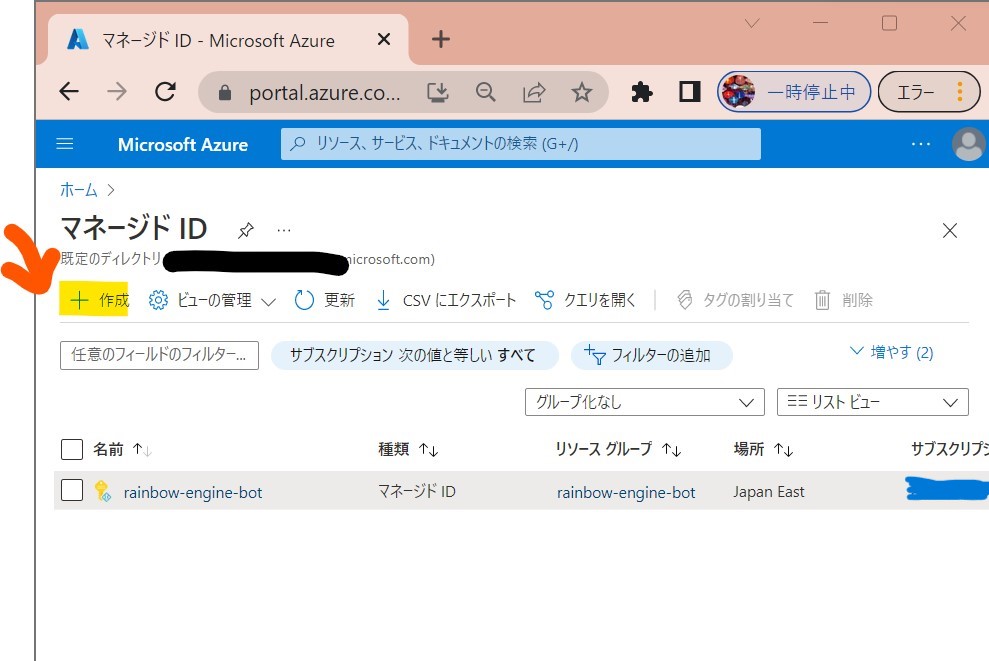
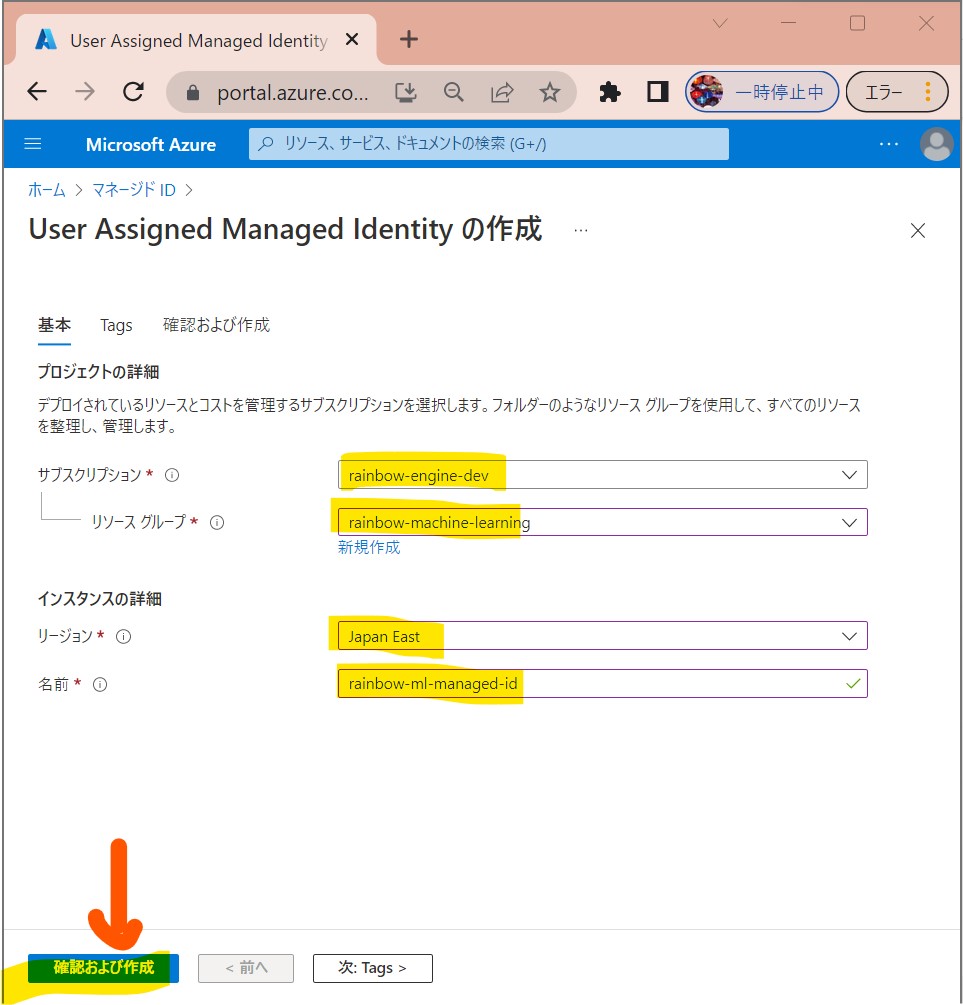
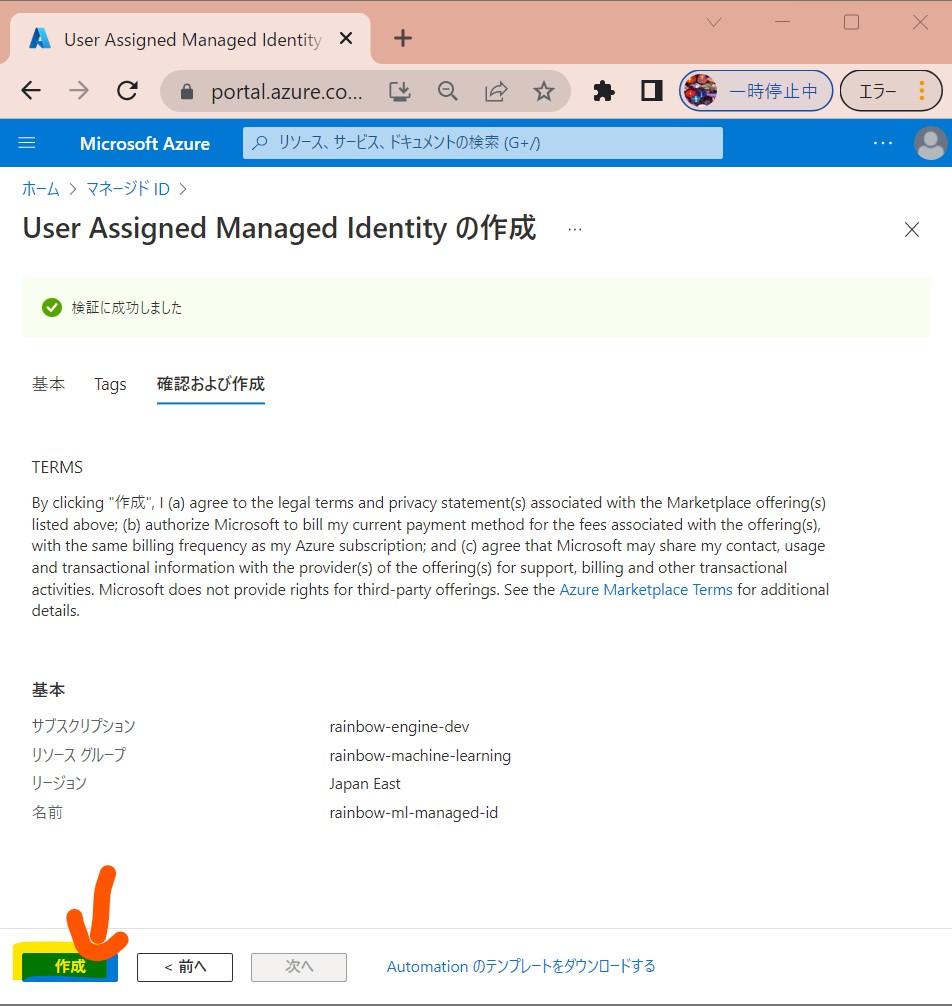
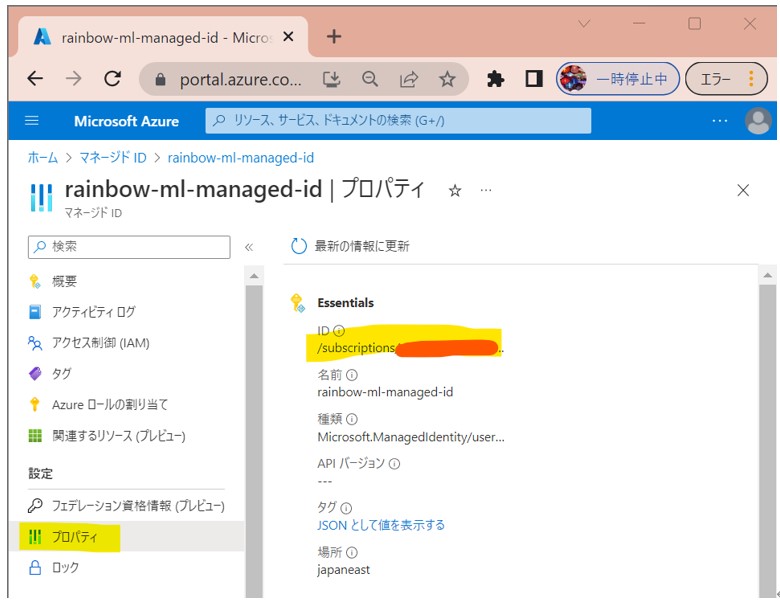
●STEP2-2:Managed Identityを持つCompute Clusterの作成
# Handle to the workspace from azure.ai.ml import MLClient # Authentication package from azure.identity import DefaultAzureCredential credential = DefaultAzureCredential()
# Get a handle to the workspace ml_client = MLClient( credential=credential, subscription_id="[ご自身のSubscription ID]", resource_group_name="rainbow-machine-learning", workspace_name="rainbow-ml-workspace", )
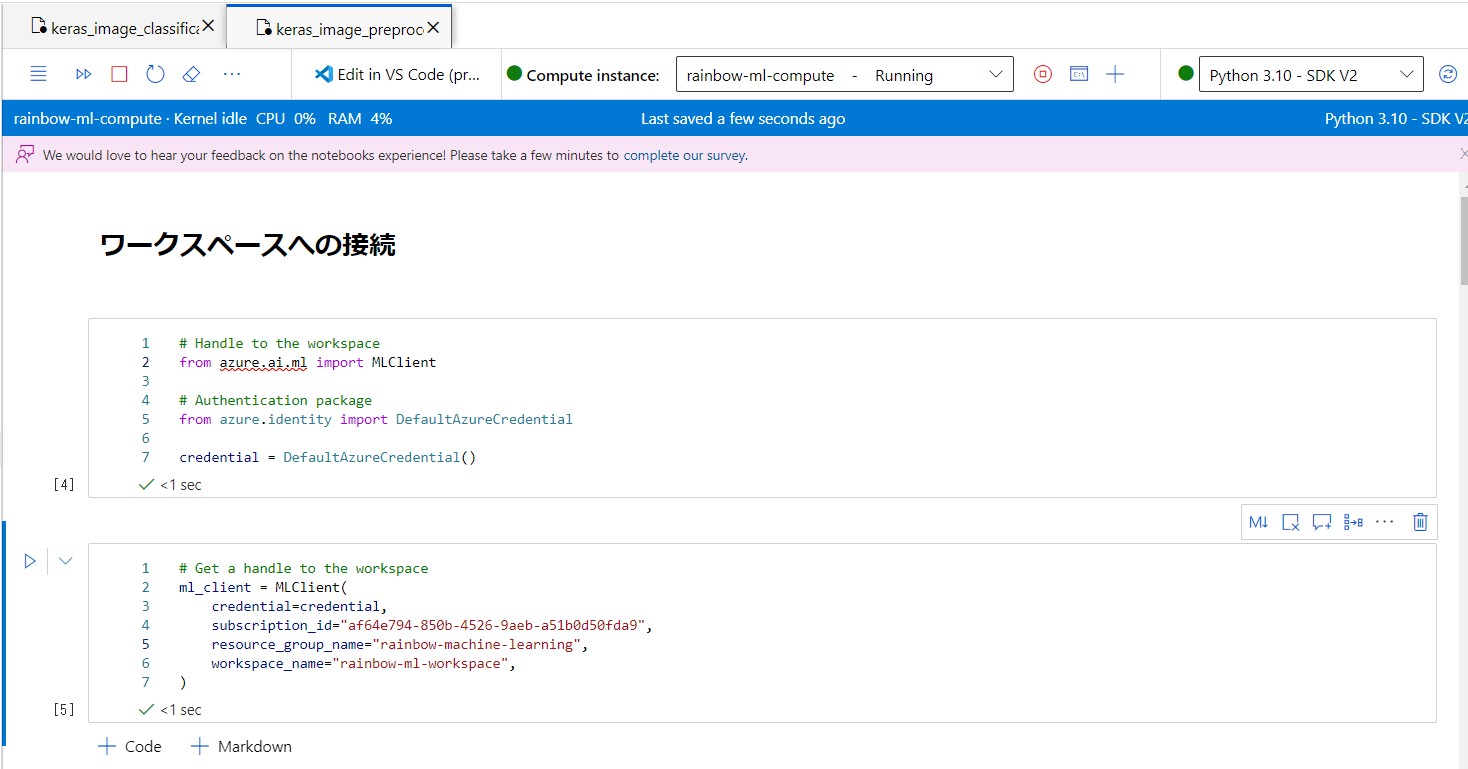
# (参考)https://learn.microsoft.com/en-us/answers/questions/1148536/cannot-access-azure-storage-file-shares-from-azure.html from azure.ai.ml.entities import ManagedIdentityConfiguration, IdentityConfiguration, AmlCompute from azure.ai.ml.constants import ManagedServiceIdentityType # Create an identity configuration from the user-assigned managed identity managed_identity = ManagedIdentityConfiguration( resource_id="/subscriptions/[ご自身のSubscription ID]/resourcegroups/rainbow-machine-learning/providers/Microsoft.ManagedIdentity/userAssignedIdentities/[ご自身のManaged IDのリソース名]" ) identity_config = IdentityConfiguration(type = ManagedServiceIdentityType.USER_ASSIGNED, user_assigned_identities=[managed_identity]) # specify aml compute name. gpu_compute_target = "gpu-cluster-rainbow2" try: ml_client.compute.get(gpu_compute_target) except Exception: print("Creating a new gpu compute target...") # Pass the identity configuration compute = AmlCompute( name=gpu_compute_target, size="Standard_NC4as_T4_v3", min_instances=0, max_instances=4, identity=identity_config ) ml_client.compute.begin_create_or_update(compute)
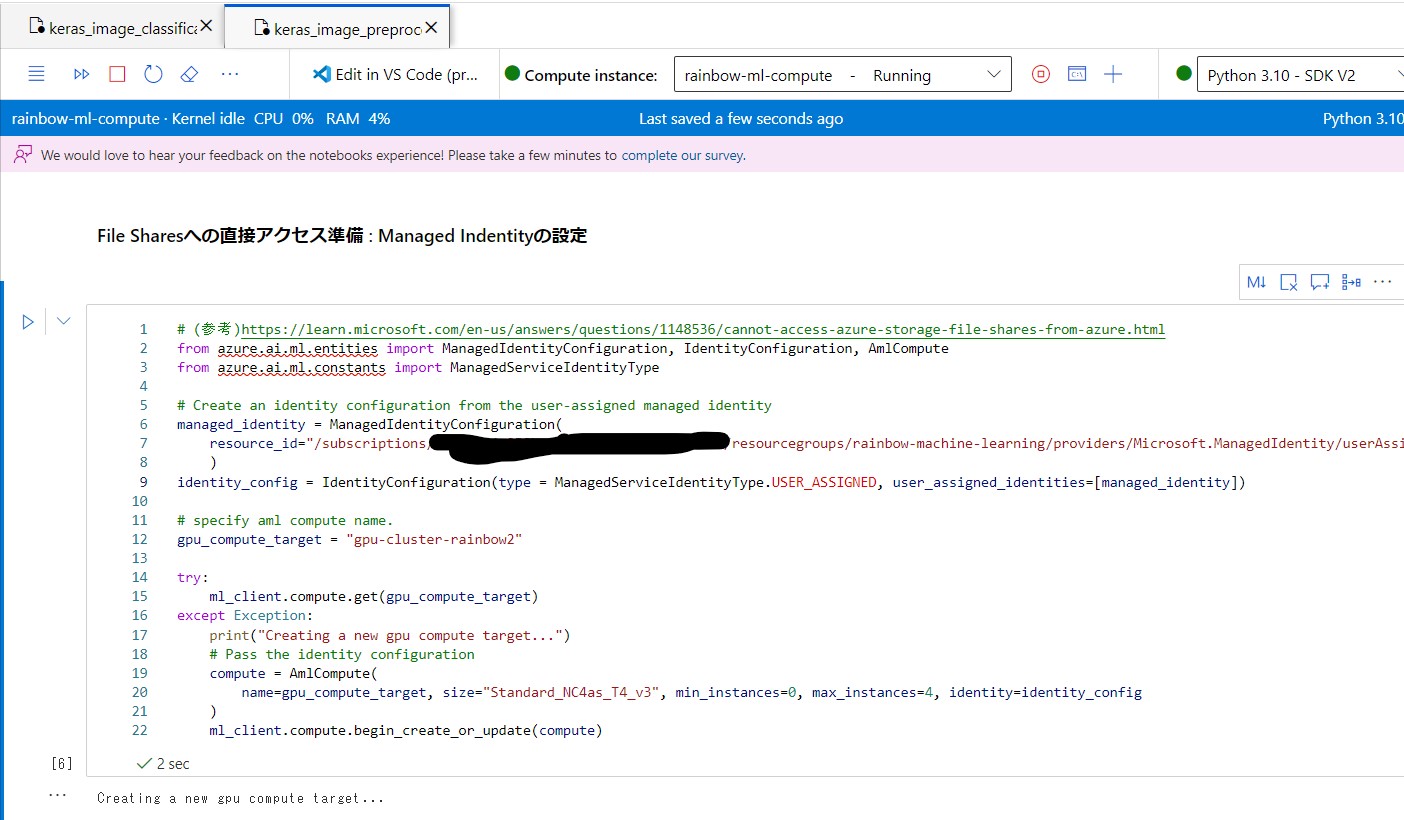
●STEP2-3:Compute Clusterの作成結果確認
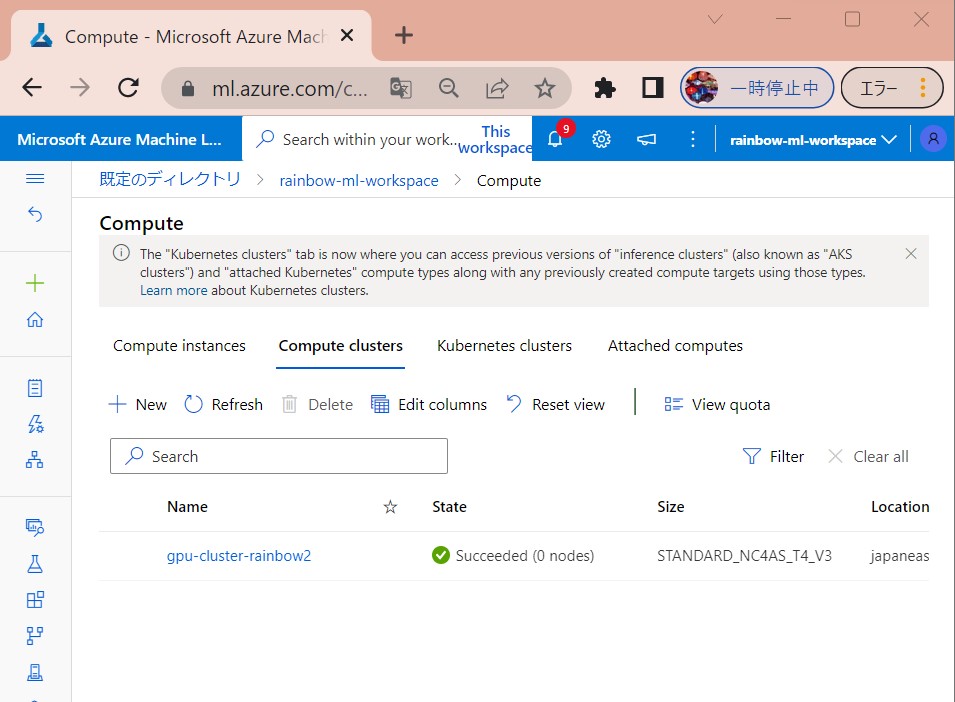
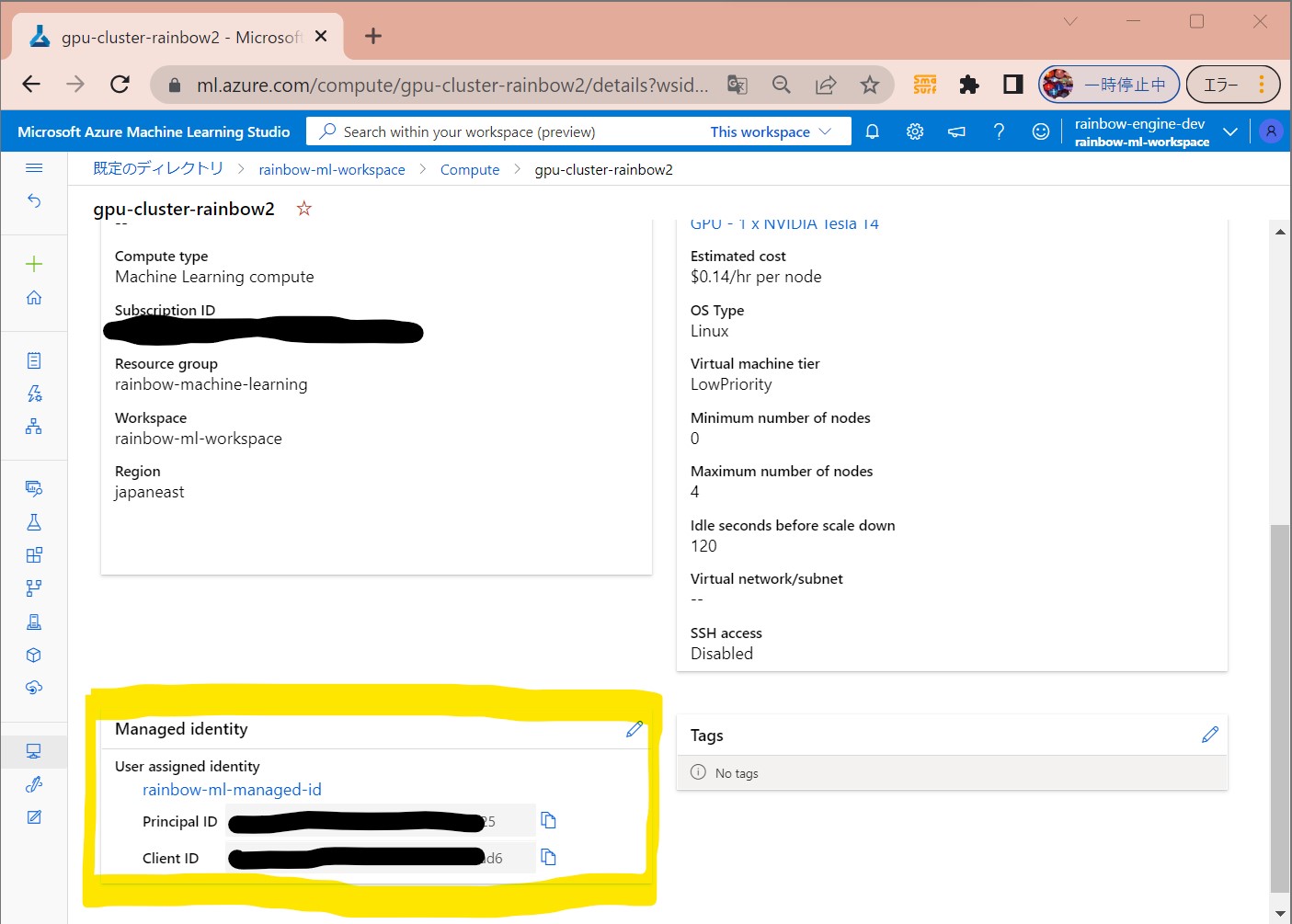
(1-3) STEP3:File Sharesへのデータアクセス処理の記述&実行
●STEP3-1:Azure Machine LearningのNotebooksの実装
from azure.ai.ml import command from azure.ai.ml import UserIdentityConfiguration from azure.ai.ml import Input gpu_compute_target = "gpu-cluster-rainbow2" custom_env_name = "keras-env" # ↓正常終了 web_path = "./data" # ジョブの最新環境を取得 job_env = ml_client.environments.get(name=custom_env_name, version=str(len(list(ml_client.environments.list(name=custom_env_name))))) # ジョブの定義 job = command( # 入力パラメータの設定 inputs=dict( data_folder=Input(type="uri_folder", path=web_path), ), # CPU/GPUリソース compute=gpu_compute_target, # 実行環境 environment=f"{job_env.name}:{job_env.version}", # 実行対象モジュールの格納先 code="./src/", # 実行対象モジュールと引数 command="python fileshare_test_get_image.py --data-folder ${{inputs.data_folder}}", experiment_name="fileshare_test_get_image", display_name="fileshare_test_get_image", ) # ジョブの実行 ml_client.jobs.create_or_update(job)
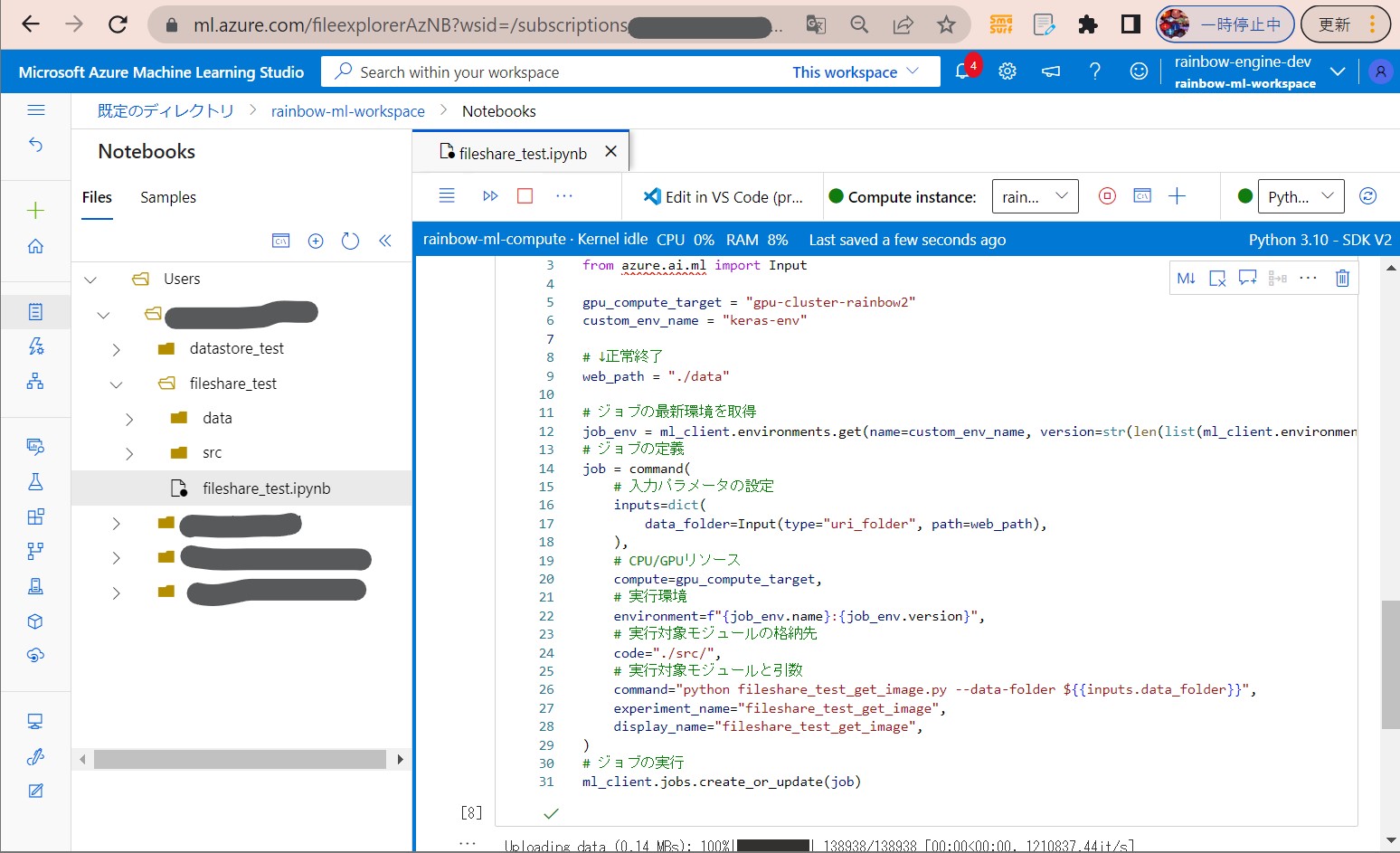
web_path = "https://rainbowmlstorage.file.core.windows.net/code-391ff5ac-6576-460f-ba4d-7e03433c68b6/Users/xxxx/datastore_test/data"
●STEP3-2:NoteBooks経由で呼び出すPythonプログラムの実装
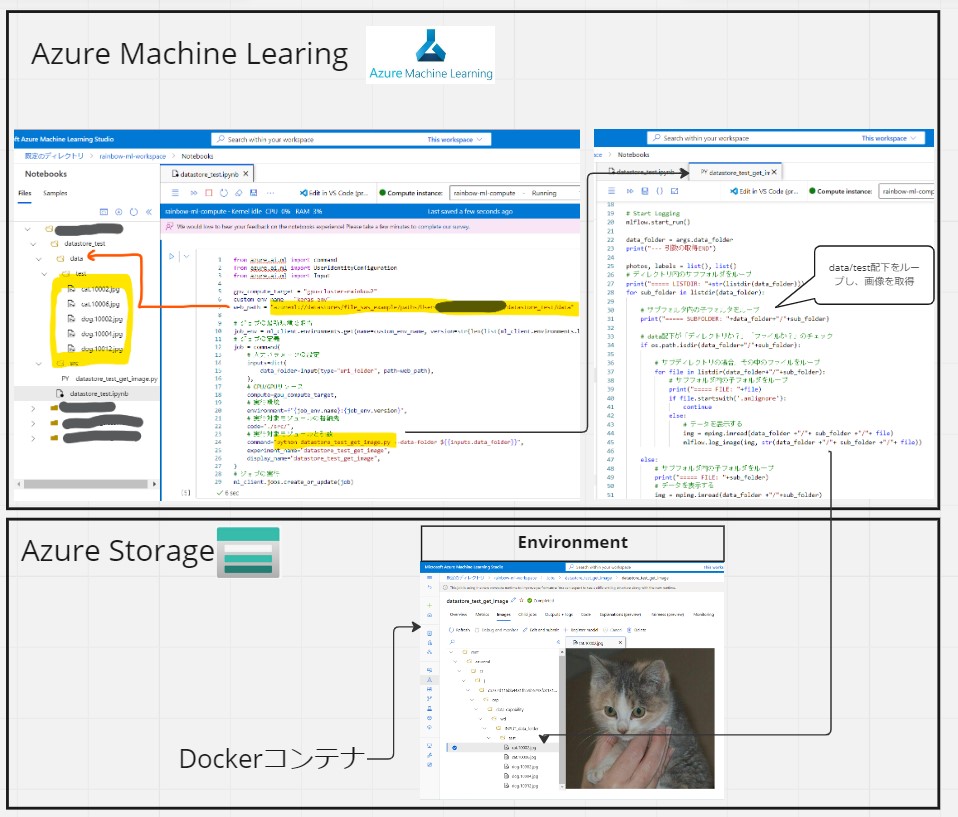
from os import listdir import os import argparse import matplotlib.image as mpimg import mlflow print("--- 引数の取得START") parser = argparse.ArgumentParser() # データセットの格納場所を設定 parser.add_argument( "--data-folder", type=str, dest="data_folder", default="data", help="data folder mounting point", ) args = parser.parse_args() # Start Logging mlflow.start_run() data_folder = args.data_folder print("--- 引数の取得END") photos, labels = list(), list() # ディレクトリ内のサブフォルダをループ print("===== LISTDIR: "+str(listdir(data_folder))) for sub_folder in listdir(data_folder): # サブフォルダ内の子フォルダをループ print("===== SUBFOLDER: "+data_folder+"/"+sub_folder) # data配下が「ディレクトリか?」「ファイルか?」のチェック if os.path.isdir(data_folder+"/"+sub_folder): # サブディレクトリの場合、その中のファイルをループ for file in listdir(data_folder+"/"+sub_folder): # サブフォルダ内の子フォルダをループ print("===== FILE: "+file) if file.startswith('.amlignore'): continue else: # データを表示する img = mpimg.imread(data_folder +"/"+ sub_folder +"/"+ file) mlflow.log_image(img, str(data_folder +"/"+ sub_folder +"/"+ file)) else: # サブフォルダ内の子フォルダをループ print("===== FILE: "+sub_folder) # データを表示する img = mpimg.imread(data_folder +"/"+sub_folder) mlflow.log_image(img, str(data_folder +"/"+sub_folder)) mlflow.end_run()
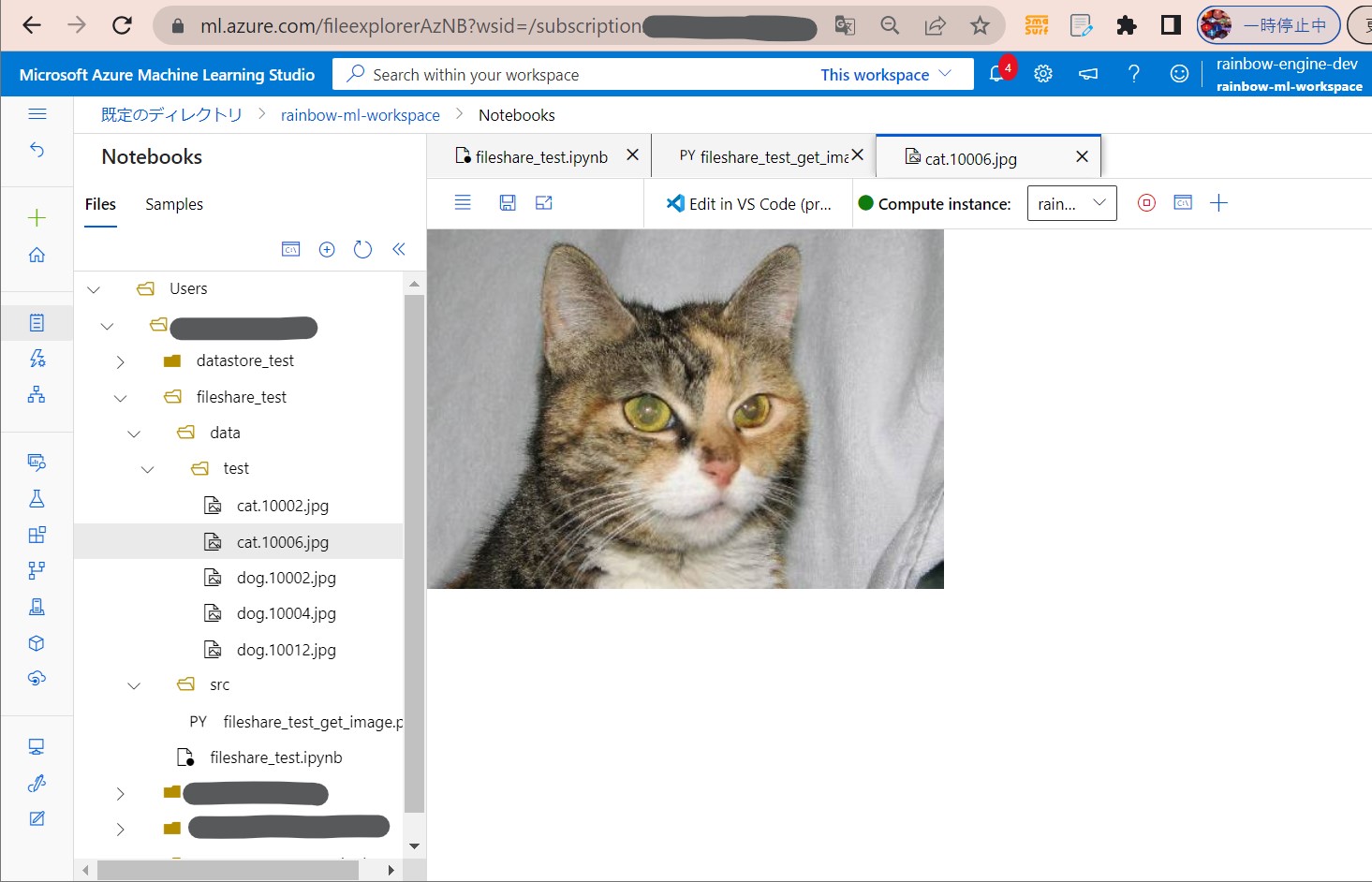
(1-4) STEP4:実行と結果確認
●STEP4-1:セルを順番に実行
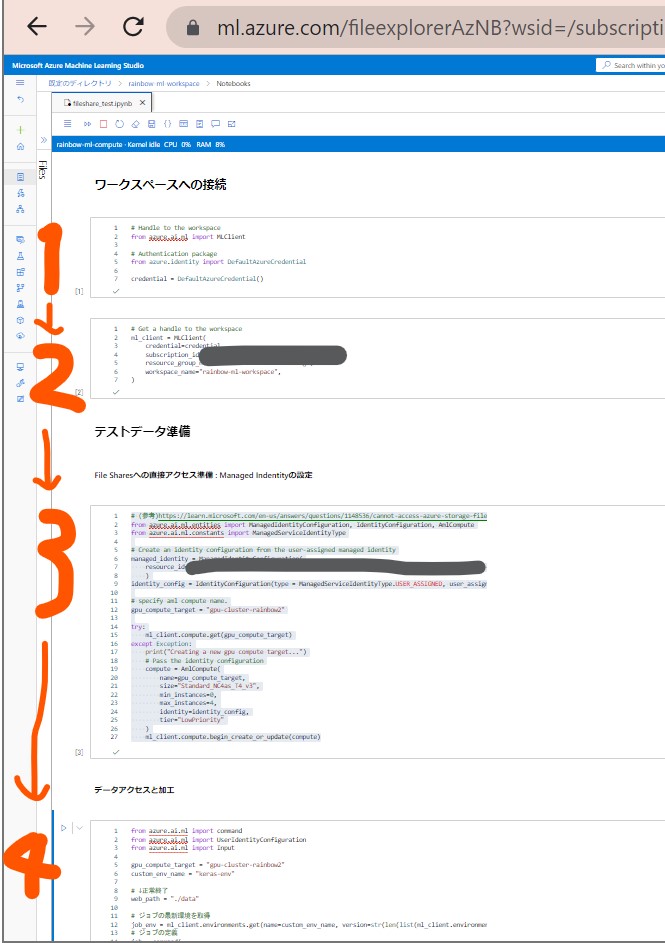
●STEP4-2:結果確認
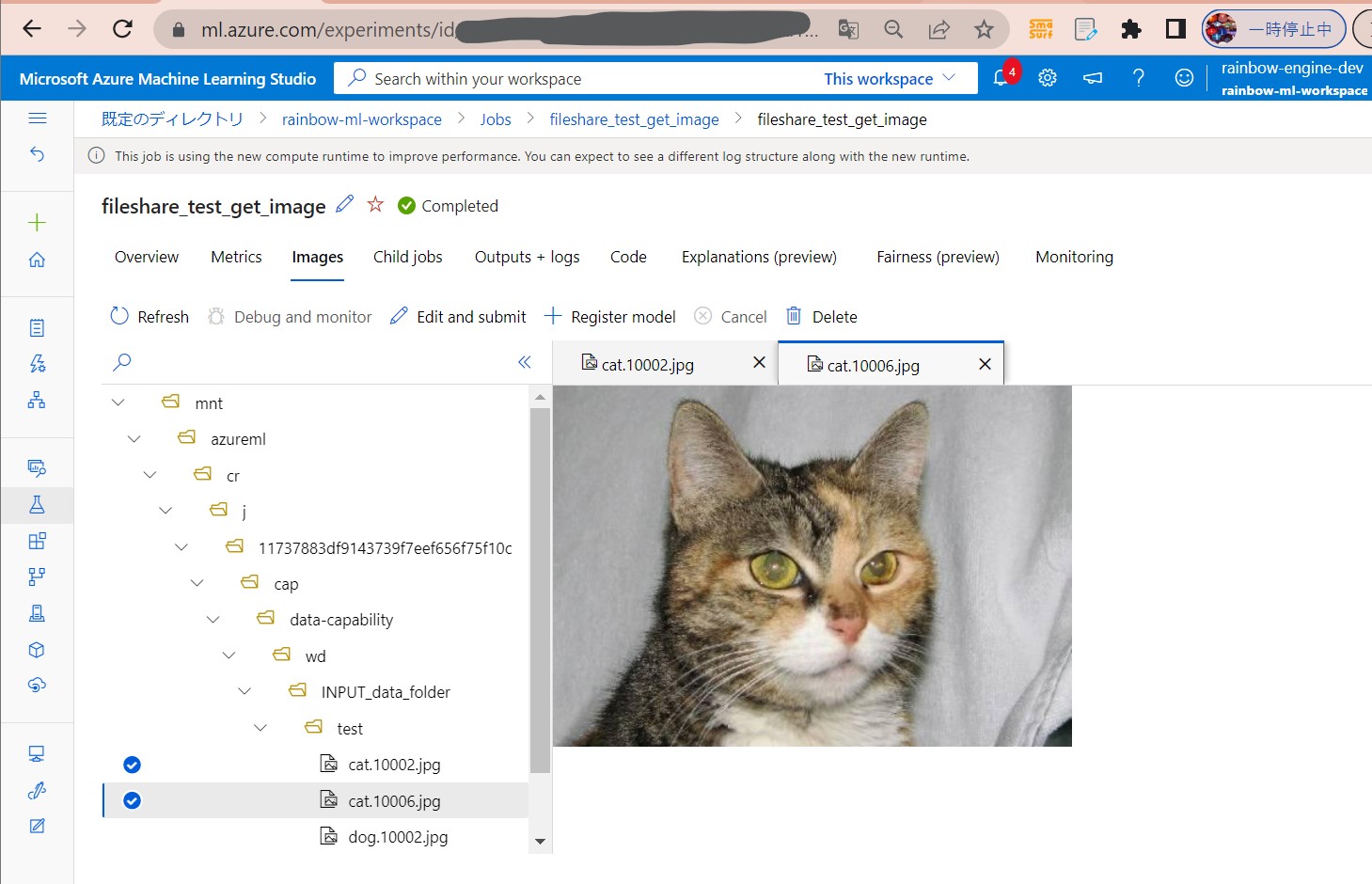